The importance of optimizing the process and maintenance of digital twins in the world of technology contributes to innovation. It helps you to stay ahead of the competition and improve the quality of your product. Surely, manufacturing with digital twins will enhance operational efficiency and offer a real-time, data-driven approach to process optimization and maintenance. To stay committed to the promise of exploring innovations in technology, digital twins are here to leave a profound impact on manufacturing. We have collected basic insights to swim into the ocean of manufacturing and maintenance. Enjoy reading!
What Are Digital Twins?
A digital twin is a virtual representation of a physical object, process, or system. It is created by collecting and integrating data from various sources, such as sensors, IoT devices, and historical records. This comprehensive digital model mimics the real-world counterpart in both form and function. Digital twins are not static; they continuously evolve as new data becomes available, making them dynamic and adaptable tools.
The Anatomy of a Digital Twin
To understand the power of digital twins in manufacturing, it’s essential to grasp their core components and functionalities:
Data Collection: Digital twins rely on a constant stream of data from physical assets and systems. Sensors and IoT devices play a crucial role in capturing real-time information about conditions, performance, and usage.
Data Integration: Collected data is integrated into a central platform, allowing for a holistic view of the asset or process. This data can include temperature, pressure, vibration, energy consumption, and more.
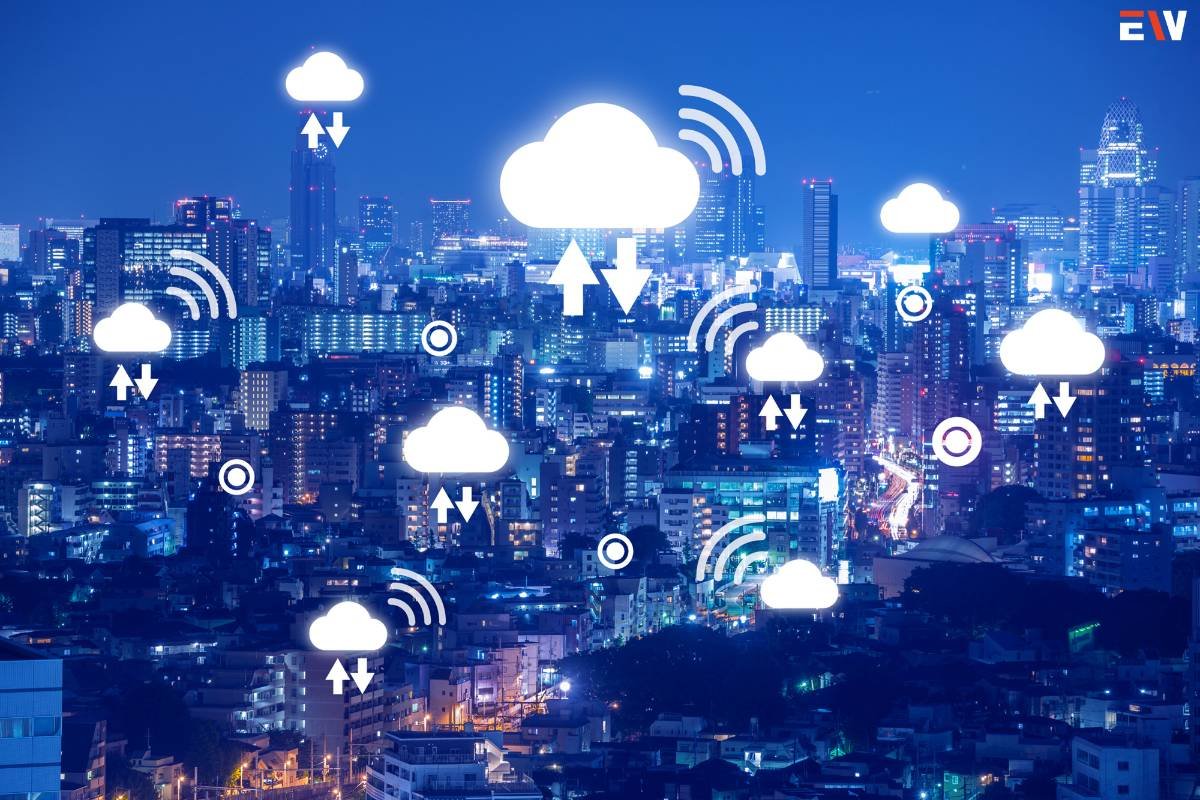
Analytics and Modeling: Advanced analytics and modeling techniques process the integrated data to create a virtual representation that mirrors the real-world asset or process. Machine learning and artificial intelligence algorithms play a vital role in this phase.
Visualization: The digital twin is visualized through 3D models, dashboards, and user interfaces, making it accessible and understandable to operators, engineers, and decision-makers.
Simulation: Digital twins enable the simulation of various scenarios and “what-if” analyses, helping manufacturers predict outcomes and optimize processes.
Applications of Digital Twins in Manufacturing;
Digital twins have found applications across various manufacturing sectors, transforming operations in numerous ways:
1. Predictive Maintenance
One of the most significant advantages of digital twins is their ability to predict equipment failures before they occur. By continuously monitoring equipment performance and analyzing data, manufacturers can schedule maintenance at the most opportune times, reducing downtime and costs.
2. Process Optimization
Manufacturers can use digital twins to simulate different production scenarios, enabling the optimization of processes for efficiency, energy consumption, and resource utilization.
3. Quality Control
Digital twins can be employed to monitor product quality in real-time. Any deviation from the expected quality parameters can trigger immediate adjustments to the manufacturing process.
4. Supply Chain Management
Digital twins extend beyond the factory floor to encompass the entire supply chain. Manufacturers can optimize logistics, track shipments, and anticipate delays or disruptions.
5. Product Development
During the product design phase, digital twins enable engineers to simulate how a product will perform in the real world. This reduces the need for physical prototypes and accelerates the design process.
Case Study: GE’s Digital Wind Farm
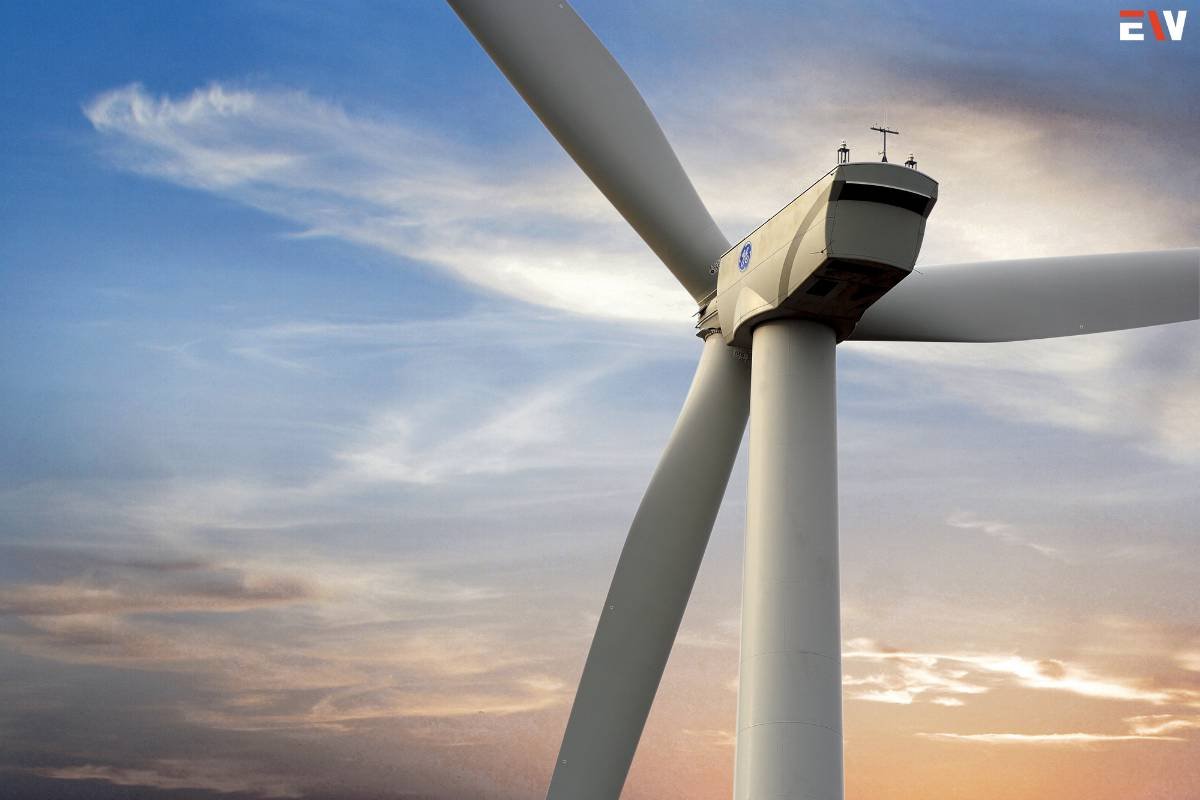
General Electric (GE) has embraced digital twins in its wind turbine manufacturing operations. The company created the Digital Wind Farm, a comprehensive digital twin of a wind farm that combines data analytics, software, and hardware to optimize energy production. By continuously monitoring the performance of individual turbines and the wind farm as a whole, GE can predict and prevent equipment failures, optimize energy output, and reduce operational costs. This innovative approach has resulted in significant improvements in wind farm efficiency and reliability.
Challenges and Considerations;
While digital twins offer substantial benefits, implementing them in manufacturing is not without challenges:
Data Privacy and Security: Collecting and storing vast amounts of data raises concerns about privacy and security. Manufacturers must ensure that data is protected against breaches and unauthorized access.
Integration Complexity: Integrating digital twin technology into existing manufacturing systems can be complex and costly. Compatibility issues and the need for new hardware and software may arise.
Data Quality: The accuracy and reliability of data are paramount. Inaccurate or incomplete data can lead to incorrect simulations and decisions.
Skills and Training: Manufacturers must invest in training their workforce to effectively use digital twins and interpret the insights they provide.
Scaling Digital Twins for Manufacturing;
Manufacturers seeking to harness the power of digital twins should follow a structured approach to ensure successful implementation:
Identify Objectives: Begin by clearly defining the goals and objectives of deploying digital twins within your manufacturing processes. Whether it’s reducing downtime, improving product quality, or optimizing resource utilization, a well-defined purpose will guide your efforts.
Data Strategy: Develop a robust data strategy that includes data collection, storage, and integration. Choose the right sensors and IoT devices to collect relevant data, and establish secure data storage practices.
Analytics Expertise: Invest in data analytics expertise. Skilled data scientists and analysts are crucial for interpreting data and deriving actionable insights from your digital twin.
Technology Selection: Choose the appropriate digital twin technology and platforms. These may include software solutions, cloud-based platforms, and hardware sensors. Ensure compatibility with your existing systems and infrastructure.
Data Quality Assurance: Implement data quality assurance measures to maintain the accuracy and reliability of the data feeding into your digital twin. Regularly monitor data sources for inconsistencies or anomalies.
Simulation and Predictive Modeling: Utilize simulation and predictive modeling capabilities to explore various scenarios and optimize processes. Assess the impact of changes before implementing them in the physical environment.
Continuous Monitoring and Feedback: Digital twins thrive on real-time data. Implement continuous monitoring to ensure that your digital twin remains up to date and provides actionable insights as conditions change.
Human-Machine Collaboration: Foster collaboration between human operators and the digital twin. Workers should be trained to interpret digital twin insights and make informed decisions based on its recommendations.
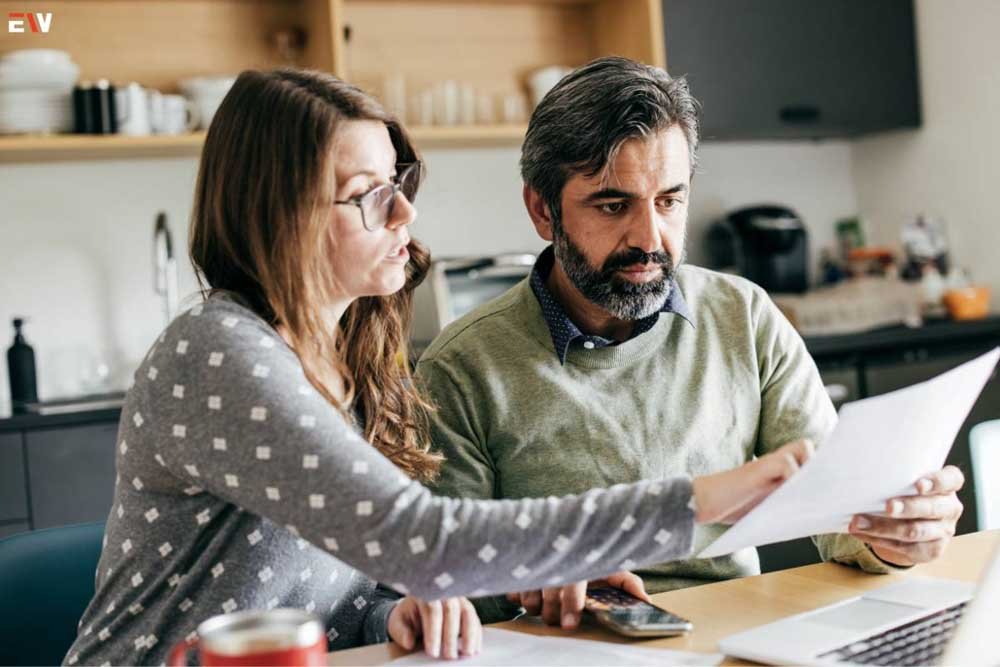
All you need to know about Servitization in Manufacturing
In an era marked by rapid technological advancements and shifting consumer preferences, the manufacturing industry is undergoing a profound transformation. Traditional manufacturing models are giving way to a more customer-centric approach known as “servitization.”
The Future of Digital Twins in Manufacturing;
As manufacturing becomes increasingly digitized and interconnected, the role of digital twins is poised to expand:
Customization and Personalization: Digital twins will play a pivotal role in enabling mass customization. Manufacturers can create personalized products by tailoring production processes through digital twin simulations.
Ecosystem Integration: Digital twins will not operate in isolation. They will be part of larger ecosystems that connect suppliers, manufacturers, and customers, facilitating end-to-end visibility and collaboration.
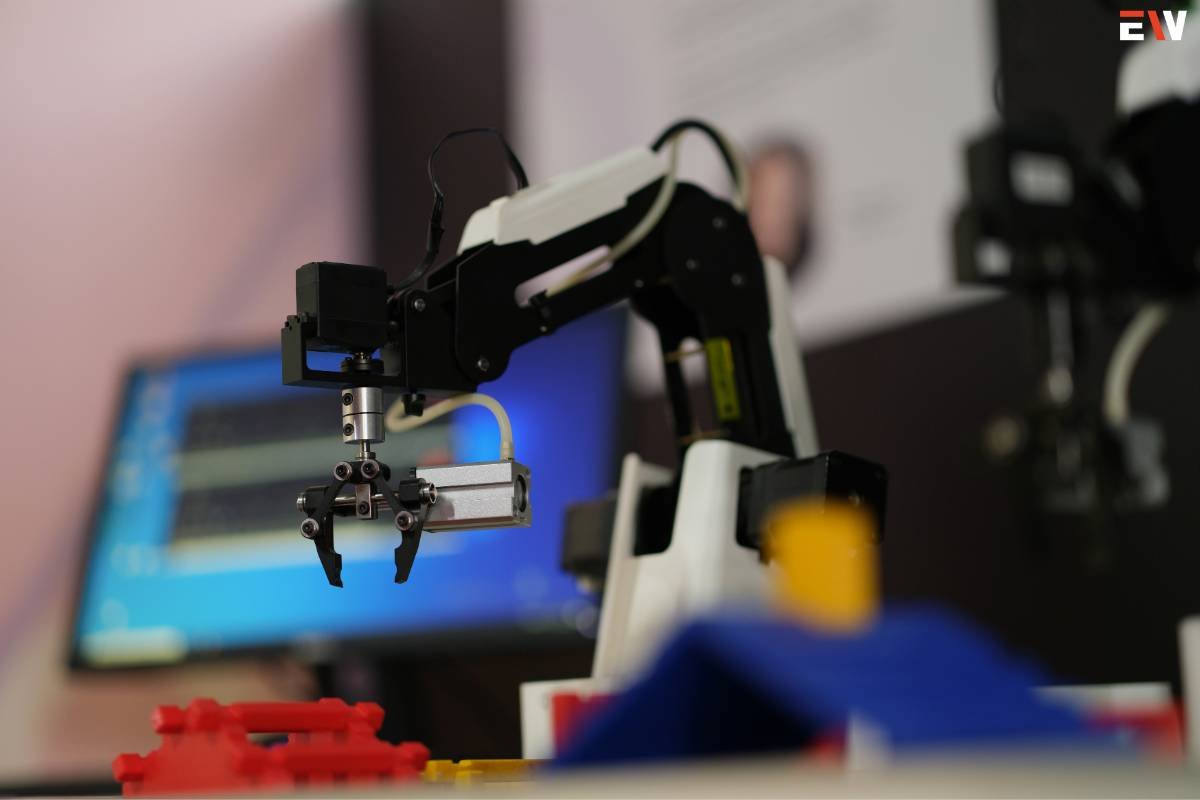
AI and Machine Learning Integration: Advanced AI and machine learning algorithms will be incorporated into digital twins, enhancing their predictive and prescriptive capabilities. These AI-driven twins will autonomously optimize processes.
Quantum Computing: As quantum computing matures, it will significantly enhance the computational power available for digital twins, enabling more complex simulations and insights.
Edge Computing: The adoption of edge computing will bring real-time processing closer to data sources, reducing latency and enabling faster decision-making by digital twins.
Conclusion
To represent digital twins you have to understand its importance in the manufacturing industry that offers real-time insights, predictive capabilities, and opportunities. It creates a virtual replica of physical assets, reduces costs, and improves product quality. Once you get the knack of variations in technology, you will master it easily. In the above-mentioned article, we believe you found the information relevant and useful to understand the importance of digital twins.