In the landscape of artificial intelligence, one term that has gained significant prominence is “Edge AI.” As the digital world continues to expand, the demand for faster and more efficient processing of data at the edge of networks has become crucial. It represents a paradigm shift in the way we approach artificial intelligence and data processing, bringing intelligence closer to the source of data generation. This article explores the concept of Edge AI, its applications, challenges, and the transformative impact it has on various industries.
Understanding Edge AI
Edge AI refers to the deployment of artificial intelligence algorithms and models on devices or local servers at the edge of a network, as opposed to relying solely on centralized cloud servers. This shift in the computing paradigm has become increasingly important, particularly with the surge in Internet of Things (IoT) devices and applications that generate massive amounts of data. By processing data locally at the edge, Edge aims to reduce latency, enhance privacy, and improve overall system efficiency.
Applications of Edge AI
1. IoT Devices and Smart Sensors
Edge is a game-changer for IoT devices and smart sensors. These devices, embedded in everyday objects, generate vast amounts of data. With Edge AI, these devices can analyze and process data locally, making real-time decisions without the need to constantly send information back to centralized servers. This is particularly beneficial in scenarios where low latency is crucial, such as autonomous vehicles, industrial automation, and healthcare monitoring systems.
2. Autonomous Systems
Edge plays a pivotal role in the development of autonomous systems. Vehicles, drones, and robots require instantaneous decision-making capabilities to navigate their environments safely. By deploying AI algorithms at the edge, these systems can process data in real time, making split-second decisions that are essential for their operation. This not only enhances safety but also reduces dependence on continuous connectivity.
3. Smart Cities
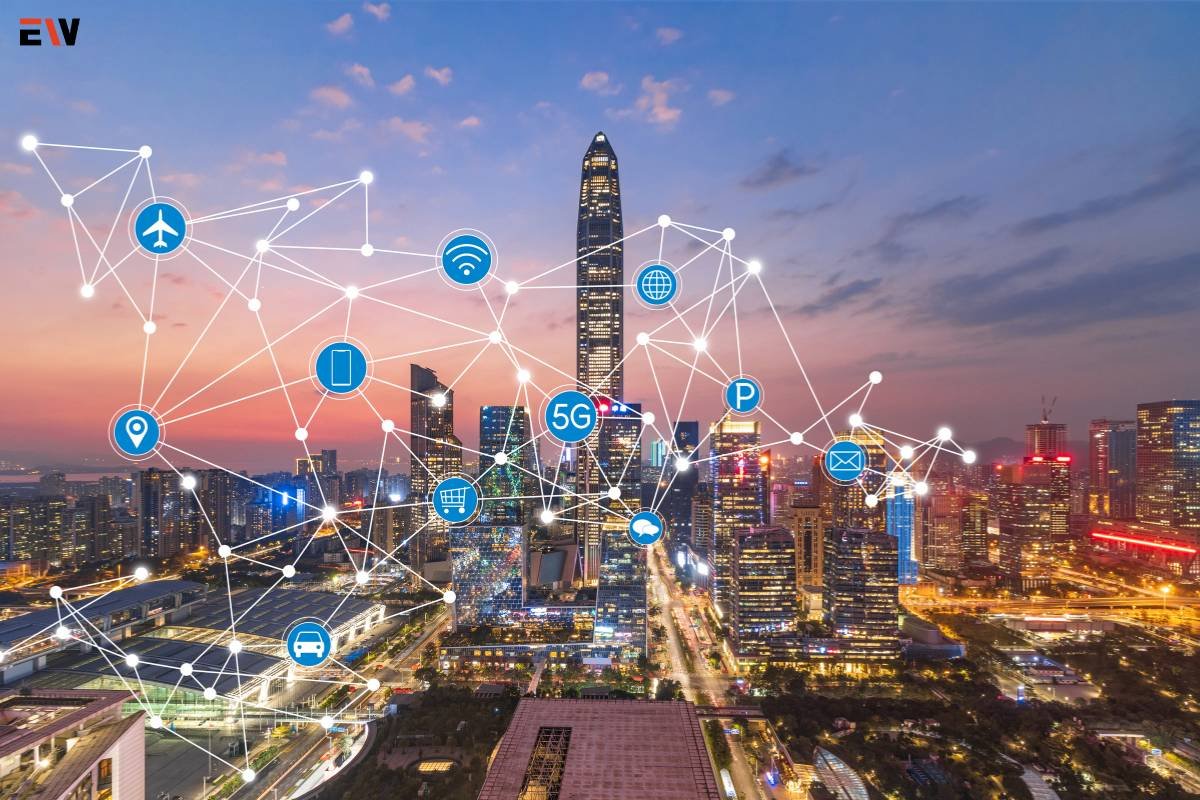
Edge contributes to the development of smart cities by enabling intelligent infrastructure. From traffic management and public safety to energy consumption optimization, Edge can process data locally, providing valuable insights for efficient city operations. This decentralized approach also enhances the security of sensitive information by minimizing data transfer to centralized servers.
4. Healthcare
In healthcare, Edge is revolutionizing patient care and diagnostics. Wearable devices and medical sensors can analyze health data locally, allowing for the timely detection of anomalies and immediate responses. This real-time processing capability is particularly critical in emergencies, where quick decision-making can be a matter of life and death.
Challenges and Considerations
While Edge AI brings a multitude of benefits, it also presents unique challenges and considerations that must be addressed for its widespread adoption.
1. Limited Resources
Edge devices, such as IoT sensors and smart cameras, often have limited processing power, memory, and energy resources. Developing AI models that can run efficiently on these devices requires careful optimization and consideration of resource constraints.
2. Security Concerns
The decentralized nature of Edge raises security concerns. Edge devices may be more susceptible to physical attacks, and securing distributed systems introduces new challenges. Implementing robust security measures, including encryption and authentication protocols, is crucial to mitigate potential risks.
3. Model Training and Updates
Training complex AI models typically requires significant computational resources, which may not be available at the edge. Moreover, updating models on edge devices poses challenges, as it must be done without disrupting the device’s primary function. Strategies for efficient model deployment, training, and updates need to be developed to ensure the longevity and relevance of Edge AI solutions.
4. Interoperability
The diversity of edge devices and platforms demands interoperability standards to facilitate seamless integration and communication. A lack of standardization can hinder the scalability of Edge AI solutions, as developers may face compatibility issues when deploying models across different devices and environments.
5. Privacy Concerns
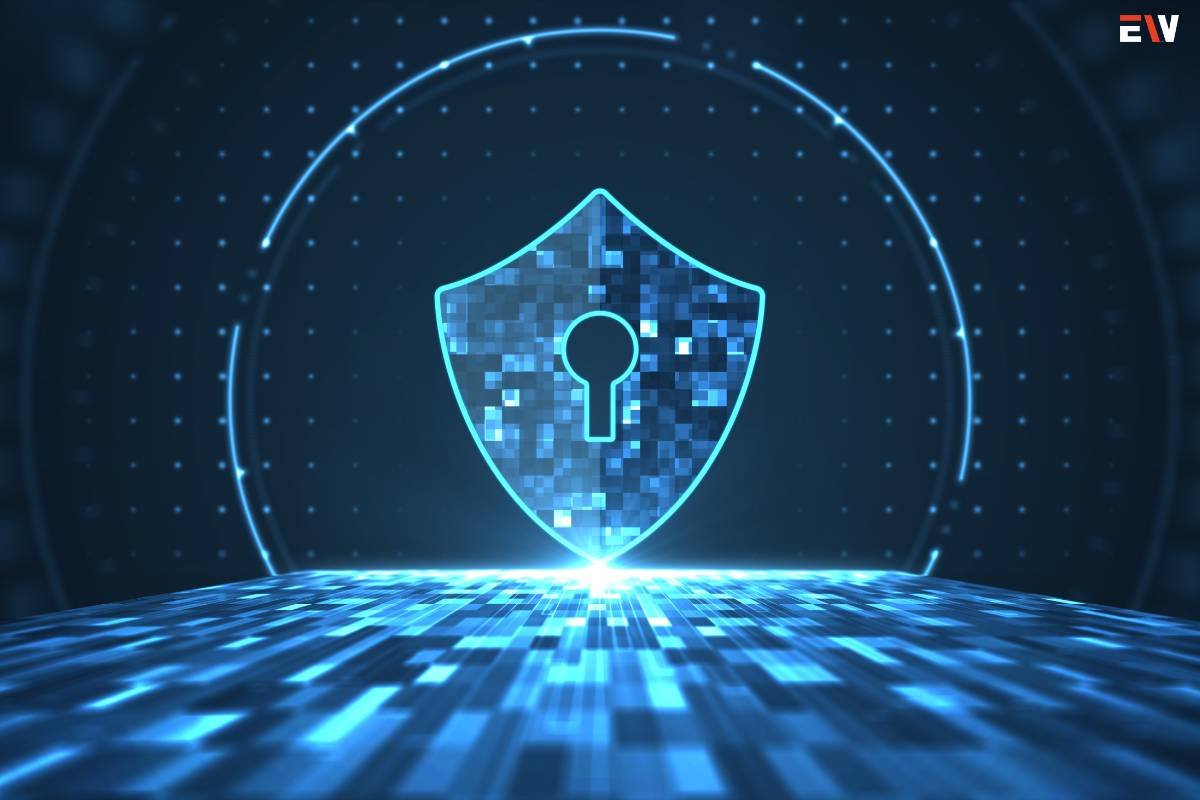
Edge processes data locally, reducing the need for constant data transfer to centralized servers. This can enhance privacy by minimizing the exposure of sensitive information. However, implementing strong privacy measures is essential to ensure that personal data is adequately protected, especially in applications such as healthcare and surveillance.
Future Outlook
The future of Edge AI holds tremendous potential for reshaping the way we interact with technology. As technology continues to advance, several trends are likely to influence its trajectory.
1. Advancements in Edge Hardware
The development of specialized hardware, such as edge processing units (EPUs) and edge inference chips, will enhance the computational capabilities of edge devices. This will allow for more complex AI models to be deployed at the edge, enabling a broader range of applications.
2. Federated Learning
Federated learning, a collaborative approach to model training without sharing raw data, aligns with its principles. This technique allows models to be trained across multiple edge devices, preserving privacy while benefiting from the collective knowledge of a distributed network.
3. 5G Connectivity:
The rollout of 5G networks will significantly impact the capabilities of this AI by providing faster and more reliable connectivity. This will be particularly beneficial for applications that require real-time communication and rapid data exchange between edge devices.
4. Hybrid Cloud-Edge Architectures:
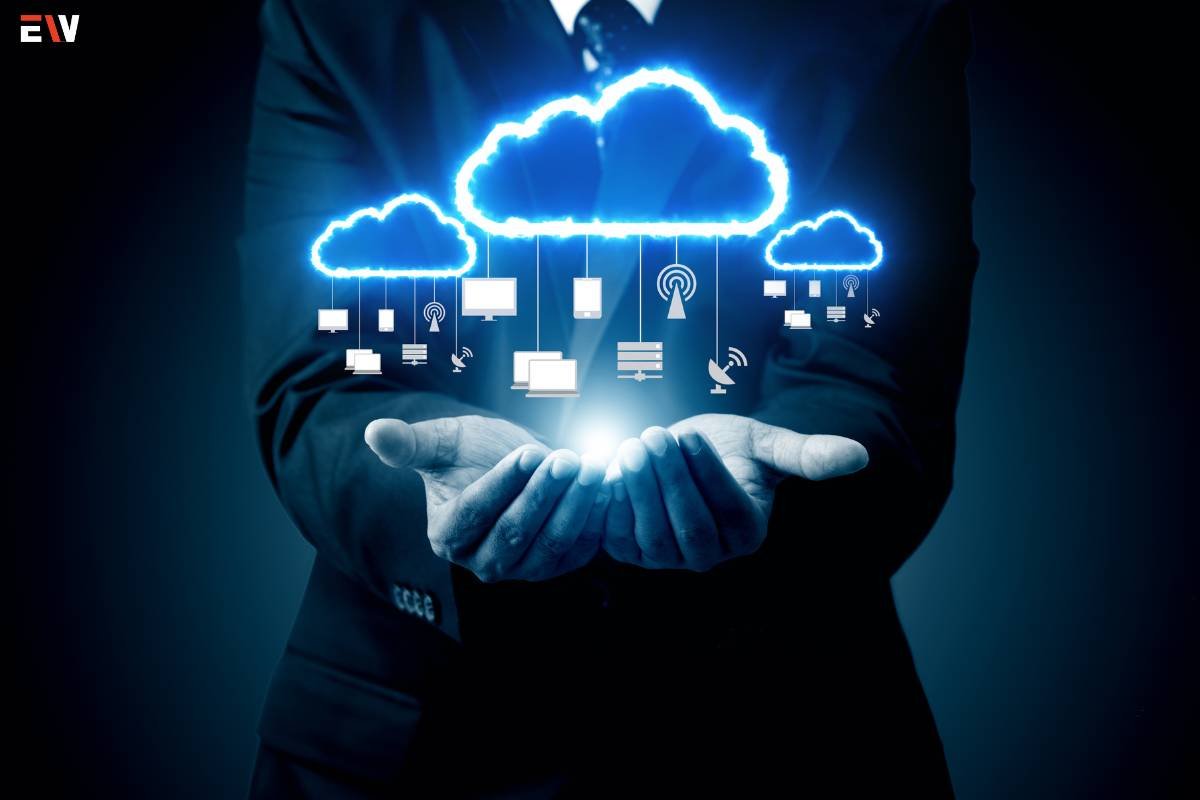
Hybrid cloud-edge architectures, combining the strengths of both cloud and edge computing, will become increasingly prevalent. This approach allows for the offloading of intensive processing tasks to the cloud while retaining the benefits of local processing at the edge.
Conclusion
Edge AI represents a paradigm shift in the field of artificial intelligence, bringing intelligence closer to the source of data generation, and its applications span various industries, from IoT devices and autonomous systems to healthcare and smart cities. While the technology offers numerous benefits, challenges such as limited resources, security concerns, and interoperability issues must be addressed for widespread adoption.